In the realm of tenant screening, determining the reliability and suitability of potential renters has long been a critical process for landlords and property managers. Traditionally, this process has relied on manual evaluation, involving tedious paperwork, reference checks, and subjective decision-making. However, with the rapid advancements in technology, a new era of tenant screening has emerged, driven by the power of machine learning.Â
By leveraging vast amounts of data and sophisticated algorithms, machine learning has the potential to revolutionize the efficiency, accuracy, and fairness of the approval policies in tenant screening.Â
In this post, we will expose the differences between traditional approval policies and machine learning-based approaches in tenant screening. We will explore the advantages and challenges associated with each method, shedding light on how machine learning algorithms can reshape the tenant screening landscape.Â
We will examine the limitations of traditional approval policies, such as human bias, lengthy processing times, and limited data insights. Conversely, we will showcase how machine learning algorithms can mitigate these challenges by automating decision-making, analyzing large datasets, and providing objective assessments of potential tenants.Â
If you want to know more about what tenant screening is and how it can help you, be sure to visit our post What is Tenant Screening and how can KBA help you?
Machine learning and traditional approval policies in tenant screening represent different approaches to evaluating tenant applications. Here’s a comparison between the two:Â
Traditional Approval Policies:Â
- Manual Process: Traditional approval policies typically involve a manual review process, where landlords or property managers manually assess tenant applications, review documents, and make decisions based on their experience and judgment.Â
- Limited Data Analysis: Traditional methods often rely on basic information provided by applicants, such as employment history, income verification, rental references, and credit checks. The analysis of these factors is typically performed individually without complex data modeling or pattern recognition.Â
- Subjectivity: Traditional approval policies can be subjective and influenced by personal biases or subjective interpretations. Decision-making may vary based on individual perspectives, which can introduce inconsistency and potential discrimination concerns.Â
- Relatively Static: Traditional policies tend to follow fixed guidelines and criteria. Updates or adjustments to the criteria may require manual changes and may not adapt to evolving rental market dynamics or tenant demographics.Â
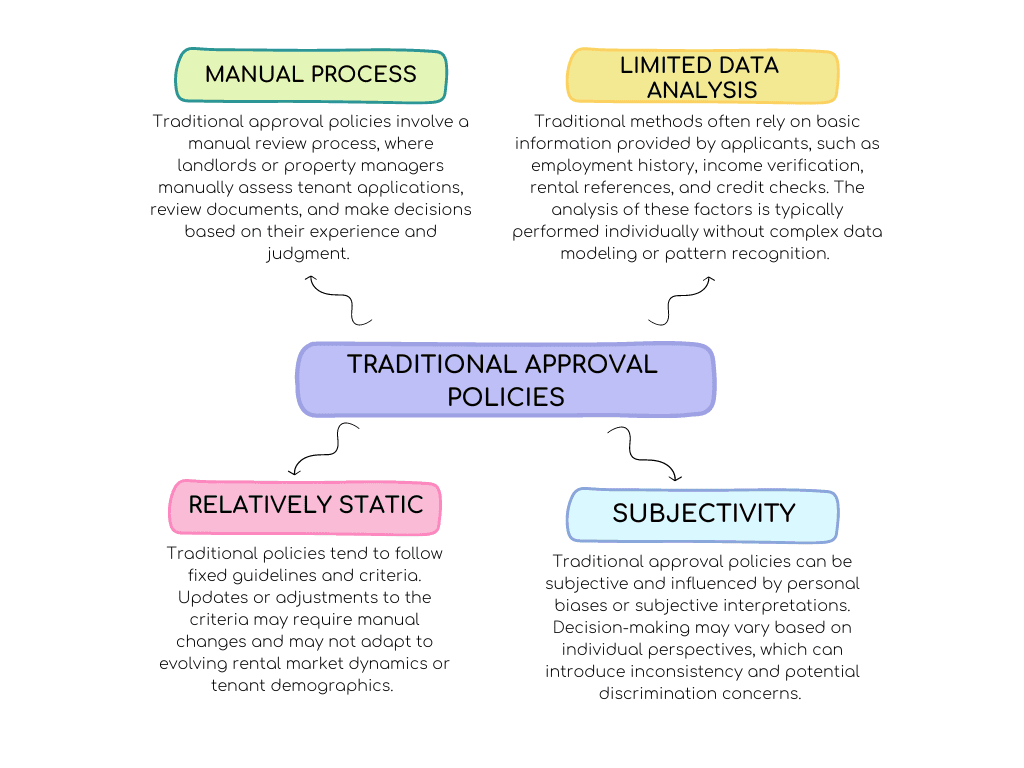
Machine Learning Approaches:Â
- Automated Data Analysis: Machine learning algorithms enable automated analysis of large datasets and capture complex patterns in tenant applications, allowing for more comprehensive assessments.Â
- Objective decision-making: Machine learning algorithms follow predefined rules and criteria, leading to more consistent and objective decision-making. Â
- Enhanced Accuracy: Machine learning algorithms leverage statistical modeling and pattern recognition to identify subtle correlations and predict future outcomes. They can uncover hidden patterns and provide more accurate predictions about tenant behavior, including payment reliability and potential lease violations.Â
- Adaptability: Machine learning models can adapt and learn from new data, allowing them to continually refine their predictions and adjust to changing circumstances. Â
- Efficiency: Machine learning algorithms can significantly speed up the screening process by automating data analysis and decision-making. This allows for quicker application processing, enabling landlords to fill vacancies more efficiently.Â
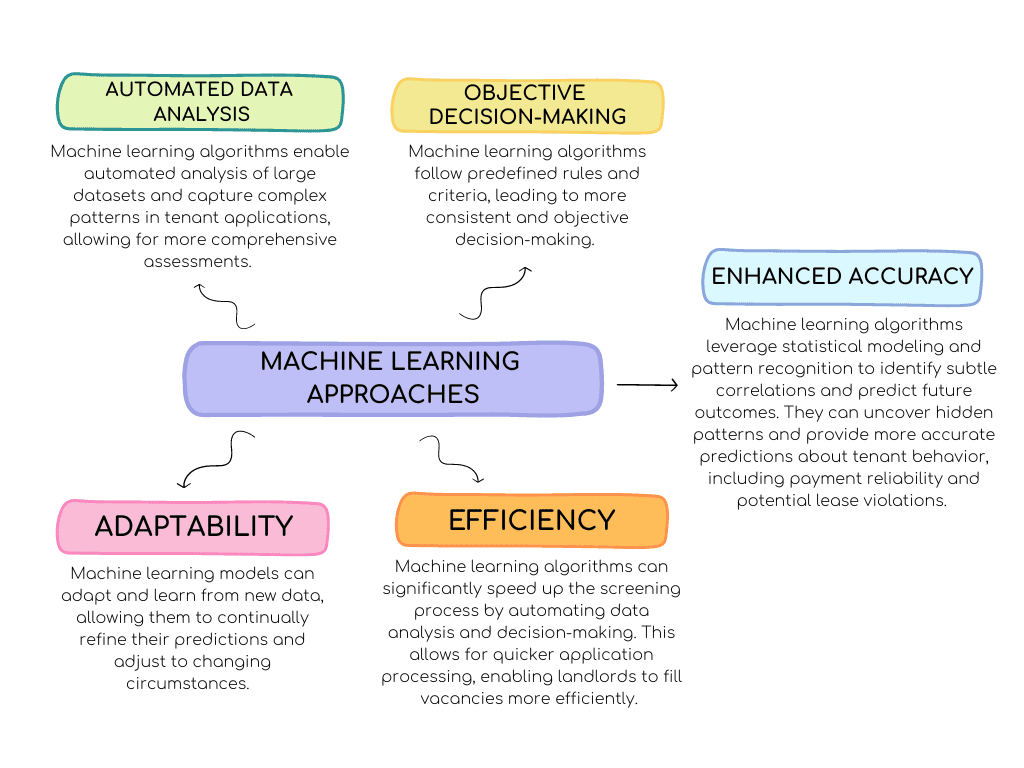
So what is better? Machine Learning or traditional approval policies?
In summary, machine learning approaches in tenant screening offer automated data analysis, objective decision-making, adaptability, enhanced accuracy, and efficiency compared to traditional approval policies. Â
The debate between machine learning and traditional approval policies in tenant screening is complex and multifaceted. While traditional methods have long been the standard, machine learning offers promising advancements and potential improvements in the screening process.Â
Machine learning algorithms can analyze vast amounts of data and identify patterns that traditional methods may overlook. Additionally, machine learning models can quickly adapt and learn from new data, constantly improving their effectiveness over time.Â
Additionally, it is important to recognize that machine learning algorithms are not a one-size-fits-all solution. They must be used in conjunction with human judgment and experience to strike a balance between automation and personalized decision making. Human oversight can bring a human touch to the tenant selection process.Â
In conclusion, the integration of machine learning into tenant screening processes has the potential to revolutionize the industry by improving accuracy, efficiency, and fairness. However, careful implementation, ongoing monitoring, and a commitment to ethical practices are essential to maximize the benefits while minimizing the risks associated with bias and discrimination.Â
How can KBA help you?
At KBA, we specialize in developing fully automated and digital decision engines for our clients. Our unique approach combines human review with machine algorithms in the following manner:Â
- The decision engine utilizes our machine learning algorithm and incorporates baseline minimum acceptance criteria defined by our clients based on their risk policies.Â
- In accordance with our clients’ risk policies, our machine learning algorithm can confirm acceptance or rejection for uncertain cases, and vice versa. This enables us to automate over 80% of the applications.Â
- For the remaining 20%, our solution includes embedded machine learning interpretation algorithms. These algorithms not only predict the risk associated with a tenant (and co-debtors) but also identify the main areas of concern. As a result, human reviewers only need to review a maximum of 20% of applications while gaining valuable insights to make final decisions.Â
Our approach has yielded significant reductions in review time and human hours for our clients. Additionally, it has contributed to lowering overall default rates and enhancing adaptability to changing market conditions.Â
By leveraging the power of automation and combining it with human expertise, we provide our clients with increased certainty and efficiency in their decision-making processes.Â
hi, I’m interested in using your API to UK and other European countries. Is that possible? If not, if I provided you data from Equifax and other credit agencies to retrain and adjust your SRS model, would that work?